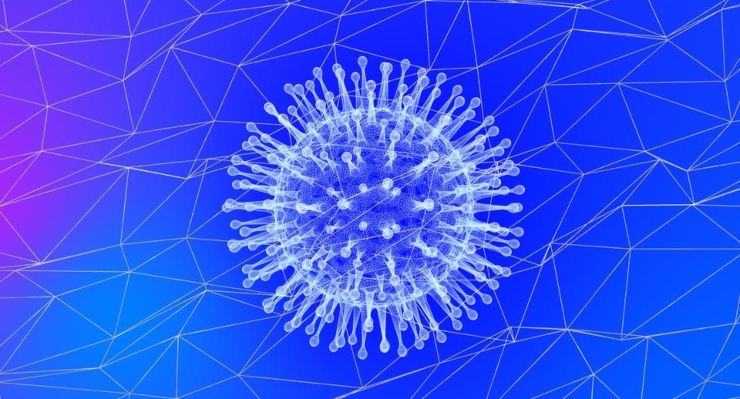
Models have become the tool of choice for telling the story of the pandemic. Problem is, in journalism they’ve landed in that murky spot where demand for future certainty meets desire for catastrophising clickbait.
They’ve fed the drift from diagnosis to prognosis. It’s a jerk back to ancient Rome, where the high priests of journalism read the entrails of the moment not so much to explain what was happening, but to predict the future.
Now that facts about “what’s happened” are ubiquitous, journalists are seeking the scoop of being the first to predict what’s going to happen — and the more awful it looks, the more likely it is to go, well, viral.
Right now there’s plenty of data to draw on. Everyone with a bit of confidence in their numeracy seems to have some data analysis. Spend any time online and you’ll know modelling has replaced Candy Crush as the online activity of choice, as people are punching in the daily publicly available COVID-19 data to understand where we’re at.
It’s been social media as a social good, providing real-time understanding of COVID and vaccination trends, with a public Twitter discussion about the appropriate application of statistical and epidemiological tools such as exponential growth, Reff, peaks and lags. Programmers have built easy-to-use mapping of exposure sites and vaccine availability from public and crowd-sourced data.
The media have climbed on board, including Casey Briggs at the ABC and the data team at Guardian Australia. People in Sydney are starting to make October dinner plans off the back of Briggs’ daily “best guesstimate” of when the state’s adults will be 70% double-vaxed.
In the UK, Financial Times’ John Burn-Murdoch has from the beginning popularised statistical tools like log graphs to simplify telling the comparative country-by-country COVID story.
Then there are the big models that make news in their own right: the now famous (or, depending on your priors, notorious) institutes like Burnet and Grattan in Melbourne and Kirby in New South Wales, and state and federal health departments. These are serious models by serious people, with serious real-world effects.
As everyone who has spent five minutes listening to any premier or prime ministerial press conference knows, it’s the predictions in the Doherty Institute modelling that has determined that sacred text of Australia’s reopening — the national plan. (Although, like lots of sacred texts, it seems open to multiple readings.)
But here’s the thing: “All models are wrong,” as scientists (social and otherwise) have recognised since British statistician George Box acknowledged this bitter truth back in the 1970s. Of course, he also added: “Some are useful.” It’s the nuanced take on Mark Twain’s more dismissive “lies, damned lies and statistics”.
Models that explain the world as it is right now are not so much wrong as they are partial — simplified. In churning real-time data through historically determined ways in which the world sorta kinda works, they provide a useful tool for painting the present.
That makes them useful, too, in providing context and depth to the core journalistic task of writing the first draft of history, of telling “what just happened”.
But models are inherently less right — potentially more wrong — in predicting what happens next. As each future input becomes fuzzier, with outcomes and interactions more variable, they take on an uncertainty that should lead journalists to tread with caution.
It’s not the modellers’ fault. Models are rendered complex by that hardest of inputs to wrangle — changing human behaviour under rapidly changing circumstances. Particularly, as in this pandemic, when the models themselves can act to create — or undermine — their own reality.
Proponents of nudge theory use predictive models to shape behaviour (“every cigarette is doing you damage”). On the other hand, modelling that suggests the need for continued hard lockdowns can, depending on the circumstances, encourage or undermine the community compliance on which the lockdowns depend.
Governments, of course, love modelling. They use its predictive power to underpin the 21st century political marketing strategy of choice — “there is no alternative” — whether it’s models of China to justify nuclear submarines or ICU beds to drive vaccination.
Journalists need to take models for what they are: not definitive statements about what will be (or gotcha claims about what went wrong) but as another politically contestable input into what is being decided right now.
I believe we need to move away from our modelling fetish and instead observe real-world actual data coming from countries that have already been down the path of mass Covid vaccinations. Canada is the stand out case for Australian observation.
Models are too easy to abuse for political ends.
….and of course endless media click bait…
100% need to base decisions on real world evidence. Professor Nikolai Petrovsky in the College of Medicine and Public Health at Flinders University and Research Director of Vaxine Pty Ltd writes: “We need to be extremely cautious about making policy decisions based on just a single model. Such models are extremely sensitive to their inputs and assumptions, and can easily provide misleading results.” Many governments based decisions on “. . . modelling results with disastrous consequences, collectively resulting in over a million avoidable deaths across those countries who did not implement any early control measures based on misplaced trust in those models. We don’t want to see a repeat of this in Australia.”. [https://www.scimex.org/newsfeed/expert-reaction-modelling-predicts-80-adult-covid-19-vaccination-wont-be-enough]
100% correct. Models need to ask the right questions though. And the right question is not how many covid cases do you have?
The ability of the hospital system to cope matters more than case numbers in our context as the goal of living with the virus is to constrain case numbers to a level that keeps hospitals afloat and a more robust hospital system can cope with more cases than a weak one. Dr Moy of AMA has called for working backwards from hospital capacity and this makes sense to me as if have 5 beds need fewer cases than if have 5000 beds. Doherty’s model projects case numbers based on interactions between effective or not test-trace-isolate-quarantine, restrictions light-hard lockdown, and vax rates (and also the order in which people are vaxed) but it does seem to make much more sense to start with hospital capacity.
Why was Petrovsky and co’s original draft of their paper based on modelling not accepted as written and the final version significantly different to the initial version in that untested assertions based on the modelling were removed. Those assertions were picked up and used by the right wing conspiracy pushing media e.g. The Washington Times and the Murdoch Media before the paper was extensively rewritten.
A problem with the mathematical models or a problem with something else?
Petrovsky was giving feedback on a model prepared by ANU, UMel,and UWA in the article I linked. Not aware he developed his own model or that the one he was commenting on had garnered press attention or been rewritten as a consequence and would love links as curious what is going on re rewriting and also as on the face of it I would have thought the right wing would have rushed to bury a model that supported high vax rates!
The model reported by Petrovsky et al in the paper that I referred to stated in the abstract that the modelling reported (the title is In silico comparison of SARS‑CoV‑2 spike protein‑ACE2 binding affinities across species and implications for virus origin) raised “important questions as to whether the virus arose in nature by a rare chance event or whether its origins might lie elsewhere” and later “Another possibility which still cannot be excluded is that SARSCoV-2 was created by a recombination event that occurred inadvertently or consciously in a laboratory handling coronaviruses, with the new virus then accidentally released into the local human population”.
That was in early 2020. Subsequent versions of the paper (there are more than one) do not make that claim and the last version makes no reference to the origins of the virus being ‘engineered’.
Most of the reviews lately available assign a low likelihood to the possibility of a laboratory accident and do not, as far as I can determine, assign a likelihood to the virus being engineered. The suggestion that the virus was ‘engineered’ made in statements such as those made for in a media outlet notorious for rejecting the scientific consensus on climate change, ozone depletion etc raises questions about the presentation of interpretations of the results of models before the results have been peer reviewed. It should be noted that the final version of the paper (as published in Nature reports) does not present that interpretation of the results.
The supposition put forward in the original paper (that had not been accepted for publication at that time) was picked up quickly by The Washington Times. The article in The Washington Times stated as a headline “Australian researchers see virus design manipulation” (May 21 2020). Quoting Petrovsky in an interview the article asserts that he stated “This, plus the fact that no corresponding virus has been found to exist in nature, leads to the possibility that COVID-19 is a human-created virus” and “It is therefore entirely plausible that the virus was created in the biosecurity facility in Wuhan by selection on cells expressing human ACE2, a laboratory that was known to be cultivating exotic bat coronaviruses at the time.” The article goes on to state ‘Mr. Petrovsky said the research team believes the quick evolution of the coronavirus and its unique ability to infect humans are either “a remarkable coincidence or a sign of human intervention.”’
Was the final version censored or did the first version and the subsequent statements make unjustifiable assertions? This example raises an important question regarding the responsibility of any person(s) presenting the results of models to have the models, the results and the interpretations independently reviewed before the results are made public because as seems to be common knowledge once the horse is through the gate the horse won’t come back.
Thanks for explain. I wasn’t aware of that paper and easy to see why it was picked up on – and distorted. It does raise important and vexxing questions and beyond peer review which was his role in the article I linked. Given our current social climate should or shouldn’t researchers take account how their work may or not be used politically? Assuming the final version backed off/reworded the possibility of engineering the decision seems to have been to abandon a scientifically neutral statement for sake of its political consequences. I disapprove in theory despite I may also may have decided in favor of social good. Very vexxed.
Only disagree with last sentence, robust models are precisely not politically contestable. It is when they are cherry picked or selected to suit political arguments that their usefulness disappears. Of course policy makers must exercise judgement in choosing which to use and how but that is not the same as allowing someone’s political choices to have a validity in relation to the integrity of the model. The models are contestable to those with expertise in the area modelled, who can interrogate them in terms of methodology, gaps, new knowledge. Robust models will change their predictions as new items come in, this is what makes them good science.
It’s probably not intended but you could be taken as supporting a position that all knowledge is equal and relative and choices are therefore just a matter of opinion. Conservatives are more and more drawn to this anti-science view, joining with the woo woo brigade and the world of the spin doctors. Ironically also making them in some sense heirs to Foucault. It’s bunk and in current conditions likely deadly bunk. In the pandemic the business lobby does this constantly, putting out faux science political policy positions or making faux science criticisms. Fortunately they are usually so brazenly self-interested and intellectually poor they are easily seen through. For these lobby groups it’s not health, or common wealth, it’s let’s go with what model serves the priority of making our members money.
Too right AP7.
Shame so many in the business lobby seem to ignore what economists model! While Qantus would benefit from overseas flights billions go out of the economy when it opens and economists say control of covid benefits businesses as people lock themselves down when covid is about.
Facts are simple and facts are straight
Facts are lazy and facts are late
Facts all come with points of view
Facts don’t do what I want them to
Facts just twist the truth around
Facts are living turned inside out
Facts are getting the best of them
Facts are nothing on the face of things
Facts don’t stain the furniture
Facts go out and slam the door
Facts are written all over your face
Facts continue to change their shape
Talking Heads, ‘Crosseyed and Painless’
‘Facts’ don’t come with a point of view and ‘facts’ don’t twist the truth around, people do. As much as I like Talking Heads quoting those lines doesn’t seem to make much sense here.
When trying to predict the future, the best you can do is to make an educated guess, based on whatever good quality info you can get your hands on, but try to keep other options open in case of an emergency.
“What happens if I’m wrong, compared to what do we gain if I’m right?”
Or as Spock might say, the Precautionary Principle.
Or is that Yoda the weirdo wookie?
I was unsure where this was going, and in the end was no wiser. I’m particularly taken by AP7’s point that the models provide information and should not be held to be equal to some nutters opinion.
Depending on the complexity they can be mighty useful. The comment about Casey Briggs on ABC is particularly strange given that most of Casey’s work is about showing what has happened, where, in what age groups etc. Having current data available makes decision making infinitely more likely to be correct. As far as models go though, the ones developed by Burnet and other academic groups, while intricate, are comparatively simple compared to the absolute guff that is produced by econometricians, and the effect of their models has had much greater impact on our lives over the last 30 years than these few lockdowns. These economic models are based on infinite assumptions, a thousand unknowable are just left to the side, and these economic models have been used for all manner of ill purposes, including in keeping the unemployment rate at 5%.
In the world of modelling, it is the economic models that are the ugly ones, and they have all the power. These are the models we should be eschewing. The health data modelling has been excellent. Of course it’s not reality, but these are closer to the mark than I suspect the author gives credit.
The models you refer to do sound like were contrived to conclude personal greed is great and sustainable, but most re: the pandemic say quite uniformly that health v the economy is a false dichotomy as economies depend upon control of covid.